Data has become one of the most valuable assets in modern times. As more companies rely on insights from data to drive critical business decisions, this data must be accurate, reliable, and of high quality. A study by Gartner predicts that only 20% of analytic insights will deliver business outcomes, with this other study citing poor data quality as the number one reason why the anticipated value of all business initiatives is never achieved.
Gaining insights from data is essential, but it is also crucial to understand the health of the data in your system to ensure the data is not missing, incorrectly added, or misused. That’s where data observability comes in. Data observability helps organizations manage, monitor, and detect problems in their data and data systems before they lead to “data downtimes,” i.e., periods when your data is incomplete or inaccurate.
What is Data Observability?
Data observability refers to a company’s ability to understand the state of its data and data systems completely. With good data observability, organizations get full visibility into their data pipelines. Data observability empowers teams to develop tools and processes to understand how data flows within the organization, identify data bottlenecks, and eventually prevent data downtimes and inconsistencies.
The Five Pillars of Data Observability
The pillars of data observability provide details that can accurately describe the state of the organization’s data at any given time. These five pillars make a data system observable to the highest degree when combined. According to Barr Moses – CEO of Monte Carlo Data – there are the five pillars of data observability:
- Freshness: Ensuring the data in the data systems is up to date and in sync is one of the biggest issues modern organizations face, especially with multiple and complex data sources. Having data freshness in your data observability stack helps monitor your data system for data timeline inconsistencies and ensures your organization’s data remains up to date.
- Distribution: Data accuracy is critical for building quality and reliable data systems. Distribution refers to the measure of variance in the system. If data wildly varies in the system, then there is an issue with the accuracy. The distribution pillar focuses on the quality of data produced and consumed by the data system. With distribution in your data observability stack, you can monitor your data values for inconsistencies and avoid erroneous data values being injected into your data system.
- Volume: Monitoring data volumes is essential to creating a healthy data system. Having the volume pillar in your data observability stack answers questions such as “Is my data intake meeting the estimated thresholds?” and “Is there enough data storage capacity to meet the data demands?” Keeping track of volume helps ensure data requirements are within defined limits.
- Schema: As the organization grows and new features are added to the application database, schema changes are inevitable. However, changes to the schema that aren’t well managed can introduce downtimes in your application. The schema pillar in the data observability stack ensures that database schema such as data tables, fields, columns, and names are accurate, up to date, and regularly audited.
- Lineage: Having a full picture of your data ecosystem is essential for managing and monitoring the pulse of your data system. Lineage refers to how easy it is to trace the flow of the data through our data systems. Data lineage answers questions such as “how many tables do we have?” “how are they connected?” “what external data sources are we connecting to?” Data lineage in your data observability stack combines the other four pillars into a unified view allowing you to create a blueprint of your data system.
Why Is Data Observability Important?
Data observability goes beyond monitoring and alerting; it allows organizations to understand their data systems fully and enables them to fix data problems in increasingly complex data scenarios or even prevent them in the first place.
Data observability enhances trust in data so businesses can confidently make data-driven decisions
Data insights and machine learning algorithms can be invaluable, but inaccurate and mismanaged data can have catastrophic consequences.
For example, in October 2020, Public Health England (PHE), which tallies daily new Covid-19 infections, discovered an Excel spreadsheet error that caused them to overlook 15,841 new cases between September 25 and October 2. The PHE reported that the error was caused by the Excel spreadsheet used to collect the data reaching its data limit. As a result, the number of daily new instances was far larger than initially reported, and tens of thousands of people who tested positive for Covid-19 were not contacted by the government’s “test and trace” program.
Data observability helps monitor and track situations quickly and efficiently, enabling organizations to become more confident when making decisions based on data.
Data observability helps timely delivery of quality data for business workloads
Ensuring data is readily available and in the correct format is critical for every organization. Different departments in the organization depend on quality data to carry out business operations — data engineers, data scientists, and data analysts depend on data to deliver insights and analytics. Lack of accurate quality data could result in a breakdown in business processes that can be costly.
For example, let’s imagine your organization runs an ecommerce store with multiple sources of data (sales transactions, stock quantities, user analytics) that consolidate to a data warehouse. The sales department needs sales transactions data to generate financial reports. The marketing department depends on user analytics data to effectively conduct marketing campaigns. Data scientists depend on data to train and deploy machine learning models for the product recommendation engine. If one of the data sources is out of sync or incorrect, it could harm the different aspects of the business.
Data observability ensures the quality, reliability, and consistency of data in the data pipeline by giving organizations a 360-degree view of their data ecosystem, allowing them to drill down and resolve issues that can cause a breakdown in your data pipeline.
Data observability helps you discover and resolve data issues before they affect the business
One of the biggest flaws with pure monitoring systems is they only check for “metrics” or unusual conditions you anticipate or are already aware of. But what about cases you didn’t see coming?
In 2014, Amsterdam’s city council lost €188 million due to a housing benefits error. The software the council used to disburse housing benefits to low-income families was programmed in cents rather than euros, which inadvertently caused the error. The software error caused families to receive significantly more than they expected. People who would typically receive €155 ended up receiving €15,500. More alarming, in this case, is that nothing in the software alerted administrators of the error.
Data observability detects situations you aren’t aware of or wouldn’t think to look for and can prevent issues before they seriously affect the business. Data observability can track relationships to specific issues and provide context and relevant information for root cause analysis and remediation.
A new stage of maturity for data
Furthermore, the rise of Data Observability products like Monte Carlo Data demonstrate that data has entered a new stage of maturity where data teams must adhere to strict Service Level Agreements (SLAs) to meet the needs of their business. Data must be fresh, accurate, traceable, and scalable with maximum uptime so businesses can effectively operationalize the data. But how does the rest of the data stack live up to the challenge?
Deliver Fresh Data With Striim
Striim provides real-time data integration and data streaming, connecting sources and targets across hybrid and multi-cloud environments. With access to granular data integration metrics via a REST API, Striim customers can ensure data delivery SLAs in centralized monitoring and observability tools.
To meet the demands of online buyers, Macy’s uses Striim to replicate inventory data with sub-second latency, scaling to peak holiday shopping workloads.
Furthermore, Striim’s automated data integration capabilities eliminates integration downtime by detecting schema changes on source databases and automatically replicating the changes to target systems or taking other actions (e.g. sending alerts to third party systems).
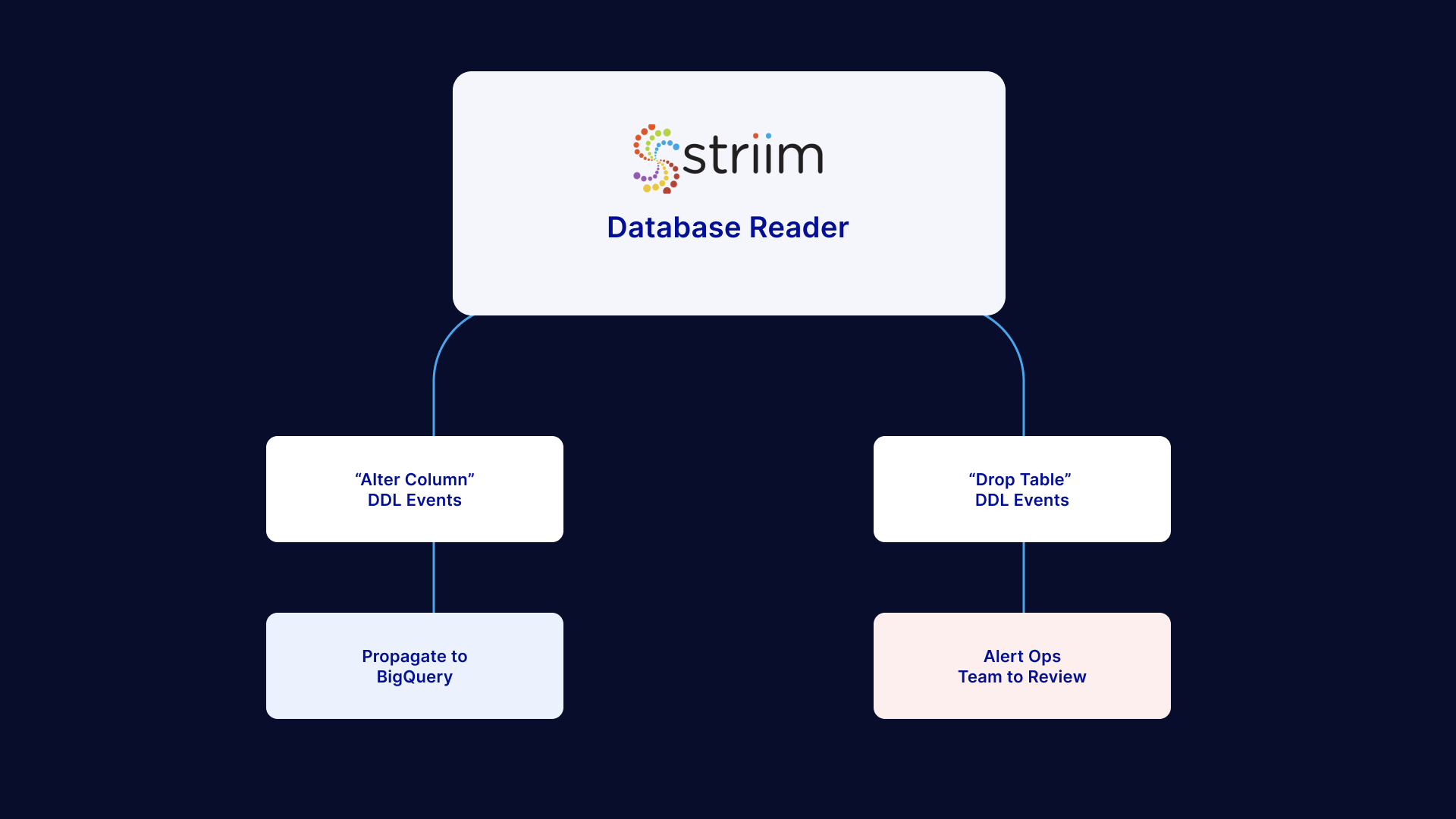
Learn more about Striim with a technical demo with one of our data integration experts or start your free trial here.