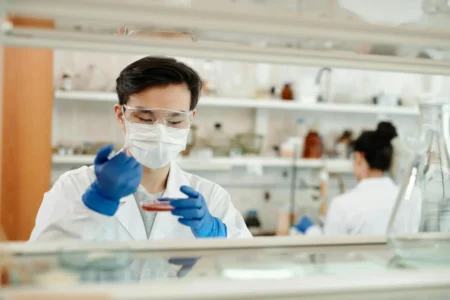
Optimizing Hospital Operations with Machine Learning in Healthcare: A Data-Driven Approach
Real-time data and machine learning are revolutionizing how hospitals operate and deliver care. By adopting a data-driven approach to hospital optimization, healthcare professionals’ jobs become