In today’s world, analyzing data as it’s generated is a key commercial requirement. A survey by Oxford Economics found that only 42% of executives can use data for decision-making. The lack of data availability impedes an organization’s ability to use data to improve customer experiences and internal operations.
A modern real-time analytics tool can empower businesses to make faster, well-informed, and more accurate decisions. By acting immediately on the information your data sources generate, these tools can improve the efficiency of your business operations. According to McKinsey, organizations adopting data analytics can improve their operating margins by 60%. However, choosing a real-time analytics tool can be tricky because one might not know what type of criteria to use while looking for a tool.
Your decision-making has a major impact on your organization’s operations for a long time, so you need a reliable real-time analytics tool to support it. Here are some considerations that can help in that regard.
Non-intrusive collection of data from operational sources
Modern businesses often deal with data streams — the continuous flow of data generated by a wide range of operational data systems. For example, a retailer can analyze transactions in real time to see if there’s any insight that indicates credit card fraud.
An operational data system generates data related to a business’ day-to-day operations. This can simply be inventory data for a manufacturing plant or customer purchase data for a retailer. A real-time analytics solution needs to support the collection of these streams from their sources.
For most businesses, data isn’t collected from a single source. Data are split into different sources based on different departments and their teams. Before performing real-time analytics on these data, you have to consolidate them into a single source of data.
It’s also important to look into the change data capture (CDC) approach your tool uses to collect and update data. If it uses triggers, then it can affect the performance of the source system by requiring multiple write operations to the source system. This interference to the system’s performance can be removed by using a tool that supports log-based CDC.
Unlike other CDC approaches, log-based CDC doesn’t affect the source system’s performance as it doesn’t scan operational tables. For this reason, you need a real-time analytics solution that provides non-intrusive data collection from multiple operational sources.
Pre-built data connectors to get real-time data from multiple sources
A data connector is a software or process that can transfer data from a data source to a destination. For example, if you are looking to collect real-time data about customer metrics (e.g., customer effort score) and analyze them to improve your customer experiences, then you need a data connector to collect that data from your CRM and send them to a data warehouse. Over time, your data engineers can spend a lot of their time working on custom data connectors.
As an organization scales up, there comes a time when it becomes hard to manage data extraction from sources to the data warehouse. That’s because it also exponentially increases the number of required custom connectors, which increases the burden on the data engineering team. A real-time analytics solution that comes with pre-built data connectors can solve this problem.
Building connectors by yourself can take considerable time. Things don’t end with the development of connectors; you also have to maintain them. A tool with pre-built connectors can eliminate this burden. Pre-built connectors are designed to ensure that end-users can add or remove data sources with a few clicks without requiring help from specialists. Your development team can then focus their time on other critical tasks, such as creating dashboards or building machine learning algorithms.
Data freshness SLAs to build trust among business users
A service level agreement (SLA) is a contract between two parties that defines the standard of service that a vendor will deliver. SLAs are used to set realistic and measurable expectations for customers.
Similarly, you need an SLA that can set clear expectations regarding your tool’s data freshness. Data freshness is necessary because business users need to know that the data they are using to make reports or decisions aren’t outdated. A data freshness SLA is a guarantee that can help to build that trust.
Data freshness means how up-to-date or recent the data are. Data can be updated every day, every hour, or every few seconds. A data freshness SLA is a contract that an organization signs with the vendor. It describes how recent data are being delivered by the tool to the target users.
In-flight data transformations to organize information
Around 90% of the data produced every day are unstructured. To make this data organized and meaningful, organizations need to apply data transformations. For this purpose, you need to look for a tool that can transform data in motion.
Data transformation converts data from one format to another format that is compatible with the target application or system. Companies perform data transformation for different reasons, such as changing the formatting. The basic data transformations include:
- Joining: Combining data from two or more tables.
- Cleaning: Removing duplicate or incomplete values.
- Correlating: Showing a meaningful relationship between metrics.
- Filtering: Only selecting specific columns to load.
- Enriching: Enhancing information by adding context.
Often businesses fail to derive value from raw data. Data transformation can help you to extract this value by doing the following:
- Adding contextual information to your data, such as timestamps.
- Performing aggregations, such as comparing sales from two branches.
- Making your data usable while sending it to a data warehouse by changing its data types, so the latter’s users can view it in a usable format.
Streaming analytics and delivery to get real-time insights
Streaming analytics refers to analyzing data in motion in real time, which can be used to derive business insights. It relies on continuous queries for analyzing data from different sources. Examples of this streaming data include web activity logs, financial transactions, and health monitoring systems.
Streaming analytics are important because they help you to predict and identify key business events as soon as they happen, enabling you to maximize gain and minimize risk. For example, streaming analytics can be used in advertising campaigns where it can analyze user interest and clicks in real time and show sponsored ads accordingly.
Once your tool is done performing analytics, it needs to send fresh data to your target systems, which can be a CRM, ERP, or any other operational system.
Choose a real-time analytics tool that delivers all of these features
It’s no longer good enough to have a real-time analytics tool that performs some of these operations. As data increases in volume and speed across different industries, you need all the features above to get maximum value out of analytics. One of the tools that is equipped with all these features is Striim.
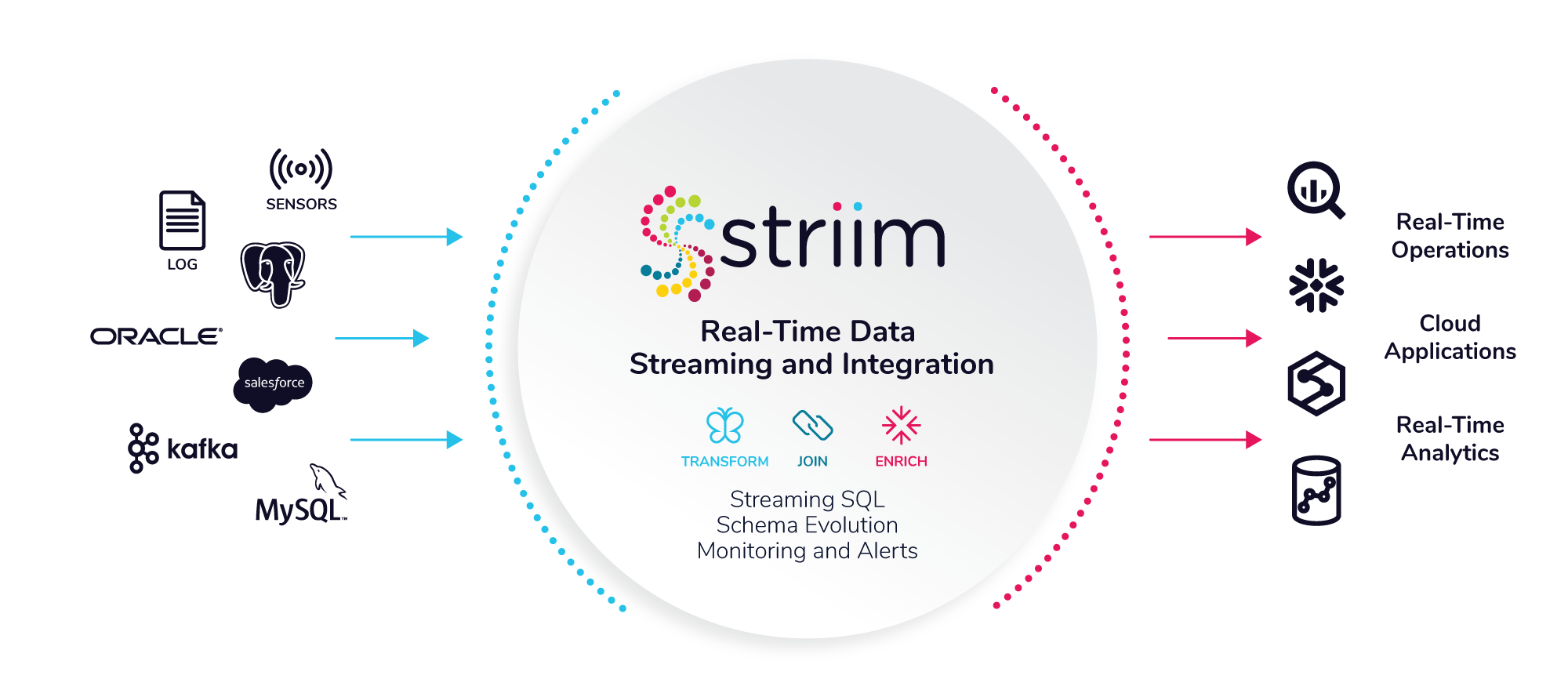
Striim supports real-time data enrichment, which other tools like Fivetran and Hevo Data don’t offer. Similarly, tools like Qlik Replicate only support a few predefined data transformations, whereas Striim allows you to not only build complex in-flight data transformations but also filter logic with SQL. Sign up for a demo right now to learn more about how Striim can help you generate valuable business insights.